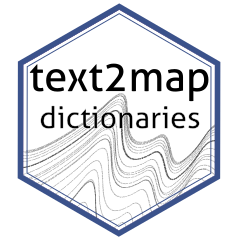
Survey of Cultural Associations from Kozlowski, Taddy, and Evans (2019)
Source:R/data.R
kte_survey.Rd
A survey of "cultural associations" between everyday objects and gender, race, and class from Kozlowski et al. (2019) used to compare with associations in word embedding models. For instance: On a scale from 0 to 100, with 0 representing very feminine and 100 representing very masculine, how would you rate a steak?" Target terms were selected from seven domains: occupations, foods, clothing, vehicles, music genres, sports, and first names
Details
As explained by the authors:
a total of 398 respondents on Amazon Mechanical Turk. The survey was fielded in two waves, the first in 2016 and the second in 2017, and was open only to Mechanical Turk users located in the United States. Although our sample cannot be said to be representative of the general population of the United States, responses to basic demographic questions indicate wide diversity in age, gender, and racial composition in the sample. To improve representativeness, we apply post-stratification weights to the sample, weighting on race (white, black, or other), education (bachelor’s degree or less), and sex (male or female). The results presented here include post-stratification weighting, but supplemental analyses available upon request show that unweighted models produce substantively similar findings.